Research & Opinion
Unveiling the Power of Generative AI: Transforming Creativity and Innovation
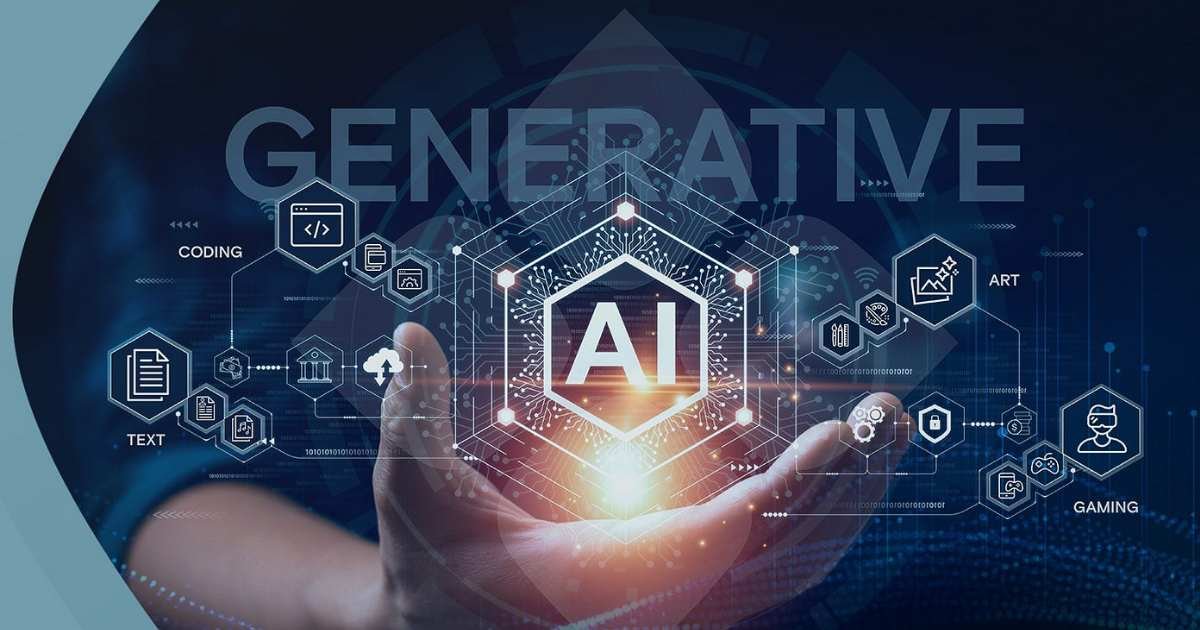
By Sharade Kamalanathan: Generative AI is a sort of artificial intelligence technology that can generate a variety of content, such as text, images, audio, and synthetic data. The recent enthusiasm surrounding Generative AI has been fueled by the ease with which new user interfaces can produce high-quality text, pictures, and movies in seconds.
The technology is not brand new. Chatbots first used generative AI in the 1960s. However, it was not until 2014, with the introduction of Generative Adversarial Networks (GANs), a form of machine learning algorithm, that Generative AI was able to construct convincingly authentic images, videos, and audits of actual people.
Transformers and The Breakdown with Large Language Models (LLMs) are two other recent advancements that have played an important role in bringing Generative AI to the public.
Transformers, for example, are a type of machine learning that allows researchers to train increasingly massive models without having to classify all of the data beforehand. New models might be trained on billions of pages of text, yielding more detailed answers. Transformers also introduced a new concept known as attentiveness, which enabled models to track word connections across pages, chapters, and books rather than just in individual phrases. And not only words. Transformers could also use their abilities to trace connections to examine code, proteins, chemicals, and DNA.
CLICK THIS LINK TO BECOME FCRF CHAPTER LEAD
ALSO READ: Join the Movement: Future Crime Research Foundation Launches State Chapters to Build a Cyber-Safe India
The fast advancement of so-called large language models (LLMs) – models with billions or even trillions of parameters – has ushered in a new era in which Generative AI models can compose engaging text, create lifelike graphics, and even create reasonably funny comedies on the fly. Furthermore, advances in multimodal AI allow teams to generate content in a variety of formats, including text, graphics, and video. This is the foundation for programs like DALL-E, which can produce images from text descriptions or text captions from photographs.
Why is Generative AI Important?
There is numerous reasons why generative AI is significant. First, it can be used to generate data that does not yet exist. This is useful for research purposes, such as when a new algorithm requires test data to train on. Second, generative AI can help to improve existing algorithms. For example, by collecting data to train new neural networks or improving deep learning architecture. Third, generative AI can be thought of as an automated machine learning technique in which a machine develops better machines. This has far-reaching ramifications beyond simply upgrading existing algorithms!
What are the Generative AI Techniques?
- Generative AI uses several strategies to identify patterns in data and generate new material:
- Generative Adversarial Networks (GANs): GANs consist of two neural networks: a generator and a discriminator network. The generator network creates new data that is identical to the source data, while the discriminator network separates the generated data from the source.
- Variational Auto-Encoders (VAE): The VAE technique allows you to encode the input into compressed code, while the decoder repeats the code’s information and stores its distribution as a much smaller dimensional representation of the compressed data.
- Transformers: Mainly trained to comprehend images or languages, transformers can also learn to classify tasks and generate content. A well-known example of this model is GPT-3 (Generative Pre-Trained Transformer – 3), which imitates cognitive attention and measures the significance of the input data parts.
How does Generative AI work – What is the process of Generative AI?
A prompt is the first thing that Generative AI uses. It could be text entered into a chat, an image, a video, or a design. Then, in response to the instruction, different AI algorithms return fresh content. Essays, problem-solving techniques, and lifelike fakes made from images or audio of real people can all be considered content. Generative AI’s early iterations required data submission through an AI or a laborious procedure. It was necessary for developers to become acquainted with specialized tools and write programs in languages like Python.
ALSO READ: Victim Of A Cyber Attack? Now Dial 1930 & 155260 To Register Complaint And Get Your Money Back
To represent and analyze content, generative AI models mix several AI techniques.
For instance, many Natural Language Processing (NLP) approaches are used to generate text. These techniques convert raw characters, such as letters, punctuation, and words, into sentences, entities, and actions. These are then represented as vectors using multiple encoding techniques. In a similar way, vectors are used to express different visual aspects from photographs. A word of caution: the training data may contain bigotry, prejudice, deceit, and puffery that these techniques can also encode.
Developers use a specific neural network to create new information in response to a prompt or question once they have decided on a representation of the world. Methods such as GANs and VAEs (a neural network with an encoder and decoder) can be used to create artificial intelligence training data, realistic human faces, or even fax copies of specific people.
Recent developments in transformers, such as Google’s Bidirectional Encoder Representations from Transformers (BERT), OpenAI’s GPT, and Google AlphaFold, have also led to the development of neural networks that are capable of producing new content in addition to encoding text, images, and proteins.
Which Generative models are available for NLP?
The following are a few examples of Generative models for NLP:
- Google’s ALBERT;
- Carnegie Mellon University’s XLNet;
- Open Ais GPT;
- Google BERT;
- Google LaMDA
What are the primary advantages that companies implementing generative AI should anticipate, and how will this enhance their competitive edge?
Businesses will undergo a radical transformation thanks to Generative AI, which has a wealth of advantages that can provide early adopters with a sizable competitive edge. Now that businesses are trying to keep ahead of the curve, let’s examine the main advantages of utilizing generative AI technologies.
- Increased Creativity and Innovation: Generative AI models are capable of producing a variety of outputs, including the creation of literature, images, music, and more. Companies can increase their creative potential and generate novel concepts and ground-breaking inventions by utilizing these talents. Generative AI can help businesses remain ahead of the competition and create original goods and solutions by enhancing human creativity.
- Enhanced Productivity and Efficiency: Businesses may automate and simplify workflows and cut down on time spent on repetitive operations by utilizing AI-generated content. Employee concentration on higher-value tasks will be made possible by this, which will eventually increase output and efficiency throughout the entire company.
- Personalized Customer Experiences: Businesses may provide their customers with experiences that are incredibly tailored to them with the use of generative AI. AI algorithms have the ability to provide content, offers, and suggestions that are specifically tailored to each user by evaluating customer data and preferences. Increased client satisfaction, loyalty, and eventually income can result from this. Characters in the film Minority Report were presented hyper-personalized and targeted advertisements, which was a fictional concept that is now becoming a reality.
- Cost-Effective Solutions: Businesses can save a lot of money over time by implementing generative AI. Businesses can cut labor expenses while preserving or even raising the quality of their output by automating repetitive processes. AI-generated content can also be less expensive than engaging experts to complete specific jobs.
- Better Decision Making: By combining enormous volumes of data and seeing patterns that human analysts would miss, Generative AI can help with data-driven decision-making. This may result in better strategy and decision-making, which will ultimately boost overall corporate performance. In reading x-rays, ECGs, and mammograms, AI programs have already surpassed 99% of physicians in accuracy.
Top risks of Generative AI
- Hallucinations: These are mistakes that artificial intelligence (AI) models frequently make because, despite their advancements, they are still not human and depend on data and training to make decisions.
- Deepfakes: A deepfake is a fake video, picture, or audio recording that mimics the appearance and likeness of another person by using generative artificial intelligence.
- Data Privacy: Since user data is frequently stored for model training, privacy is another significant risk with generative AI. It was primarily this worry that led Italy to outlaw ChatGPT, arguing that OpenAI lacked the legal right to collect user data.
- Cybersecurity: Generative AI models’ sophisticated skills, like coding, can also get into the wrong hands and pose a threat to cybersecurity.
- Copyright Concerns: Since generative AI models are trained on vast volumes of internet data, which are then utilized to produce an output, copyright is a major worry.
- Difficult to Control: Certain Generative AI models, such as GANs, exhibit behavior that is difficult to regulate and occasionally fail to provide the desired results, making it difficult to determine why.
- Pseudo Imagination: In order for generative AI algorithms to function, a sizable amount of training data is still required. GANs are not able to produce whole new objects. All they do is mix what they already know in novel ways.
- Security: Malevolent actors have the ability to employ Generative AI for fraudulent operations, identity theft, and the fabrication of false and misleading news.
To Summarize:
Businesses can gain a great deal from using Generative AI, including higher productivity, personalized client experiences, cost-effective solutions, and better decision-making. Businesses who adopt these cutting-edge technologies stand to benefit greatly from a major competitive edge, setting them up for success in a world where artificial intelligence is becoming more and more important.
About the Author:
Information Security and Data Privacy Manager, Nuvento Inc.
Master in Business Studies Human Resources Management and Finance (Gold Medallist – HRM Consistent Topper in UG and PG Certified)).
She is an IRCA certified 27001 LA, Information Security and Data Privacy Manager, Author with over four years in information security and privacy compliance. She is also a frequent speaker at industry events and her articles are published in various magazines and technical blogs.